The Invisible War: AI's Battle Against E-commerce Synthetic ID Fraud
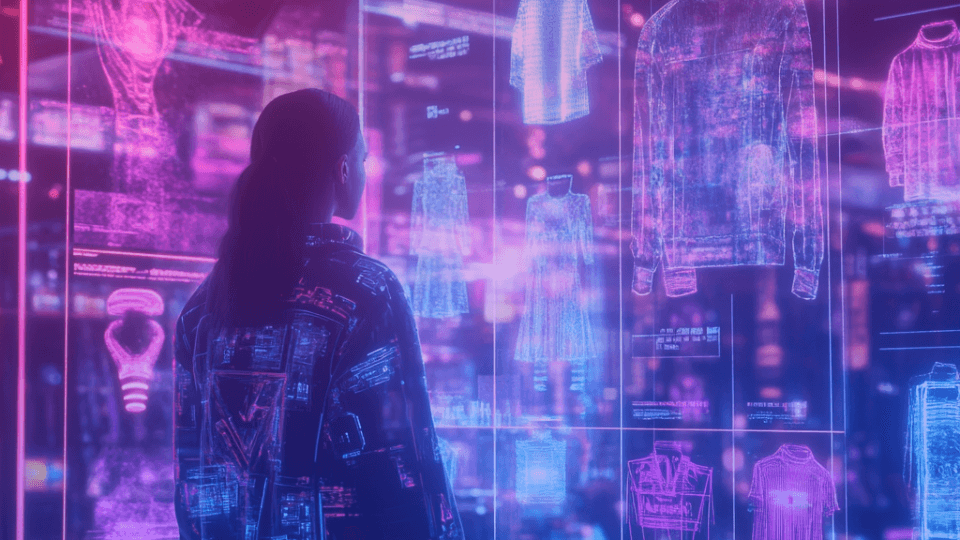
Introduction
In 2023, Amazon encountered a significant challenge: synthetic identity fraud. This sophisticated scam, powered by artificially generated identities, led to notable financial setbacks until AI-driven defense mechanisms were deployed. This situation emphasizes a crucial point: AI plays a dual role in both the creation and prevention of fraud. With the fraud rate in the APAC region climbing by 24% from 2022 to 2023, the need for cutting-edge solutions has become more apparent than ever. This dual nature of AI, serving both to innovate and to protect, sets the groundwork for an in-depth analysis of synthetic identity fraud within the e-commerce landscape.
Historical or Conceptual Foundation
The journey of identity fraud has evolved in tandem with advances in technology, shifting from manual scams to schemes powered by AI. The key milestones in this evolution include:
- Early Scams: Fraud initially depended on physical impersonation and the use of forged documents.
- Digital Shift: With the advent of the internet, phishing scams emerged, exploiting digital communications to steal personal data.
- Synthetic Identity Creation: The digital age made it easier to create synthetic identities, mixing authentic and fabricated information for fraudulent purposes.
- AI Integration: The inclusion of AI in fraud strategies and defensive measures marked a notable progression, elevating the sophistication of both attacks and counteractions.
This evolution from rudimentary forgery to AI-enabled fraud underscores an ongoing cybersecurity arms race.
The usual:
In our ongoing battle against synthetic identity fraud, AI emerges as an indispensable ally. Its deployment, however, is executed with precision and strategic insight.
- Machine Learning Algorithms: Far from being mere processors of data, these algorithms are akin to detectives of patterns. They harness the lessons learned from past incidents of fraud to fine-tune their ability to unearth future discrepancies. This process is similar to how deep learning models, which mimic the neural networks of the human brain, can detect nuanced anomalies in transaction patterns.
- Pattern Recognition: This technology goes beyond mere identification of irregularities. It delves into the complex web of data patterns to signal the presence of fraud. For instance, peculiarities in the timing of transactions or the nature of purchases could unveil activities fraught with fraud that might have previously gone unnoticed.
- Predictive Analytics: Taking a proactive stance, this approach leverages historical data to predict future fraudulent schemes. By scrutinizing the spending behaviors of new accounts, it helps in flagging those that might be potential frauds, earmarking them for closer inspection.
- Anomaly Detection: The role of anomaly detection in AI is pivotal. By establishing a baseline for 'normal' behavior, it magnifies deviations. This feature is especially critical for identifying synthetic identities, which often display transaction or account creation patterns that are far from typical.
Amid these advancements, we must not lose sight of the limitations and ethical challenges posed by AI. The danger of false positives, which could unjustly flag innocent behaviors as fraudulent, necessitates the implementation of robust safeguards and the assurance of human oversight. Moreover, the extensive data analysis required by AI to operate effectively brings forth significant privacy concerns, thereby igniting debates about the delicate balance between privacy and security.
The application of AI in detecting fraud demands a careful balance, making use of its technological capabilities while remaining vigilant of its limitations and the ethical questions it raises.
AI's Role in E-commerce Fraud Prevention: A Closer Look
As we delve deeper into the role of AI in combating e-commerce fraud, examples from industry leaders like eBay and Shopify offer valuable insights.
- eBay, leveraging machine learning, achieves up to 96% accuracy in detecting fraudulent transactions, a significant stride in eCommerce fraud prevention. This system operates 24/7, without the need for breaks, ensuring constant monitoring against fraud attacks. Despite these advancements, challenges like false positives and the necessity for manual reviews in complex cases, such as anti-money laundering (AML) checks, persist, emphasizing the blend of AI and human oversight.
- PayPal, similarly, utilizes AI and machine learning to enhance payment authorization rates and combat fraud, amid a projected $48 billion loss in e-commerce fraud in 2023.
- The double edge: The Shopify breach, involving unauthorized access by internal employees to data from nearly 200 merchants, underscores the complex nature of cybersecurity and the limitations of AI in preempting insider threats. This incident highlights a critical gap in synthetic AI fraud detection strategies: the need for adaptive, sophisticated measures that can not only detect external threats but also monitor and mitigate risks posed by insiders with access to sensitive information and systems.
Implementation factors and changes
Technological and Regulatory Navigation: Integrating AI into e-commerce platforms extends beyond the realm of technical implementation. It demands a comprehensive approach that ensures AI's functionality within a regulatory-compliant framework. Laws such as GDPR and CCPA have established stringent benchmarks for data protection, compelling AI systems to adhere to these rigorous privacy standards.
Forward-Thinking AI Implementation: The journey of AI in fraud prevention is marked by continuous learning and adaptation. Success stories like eBay's, coupled with cautionary tales from Shopify's experience, inform the strategic direction for future AI deployments. The balance between technological innovation and adherence to ethical and regulatory guidelines is crucial, influencing the future landscape of e-commerce security.
Embedding AI into your e-commerce cybersecurity posture is both a necessity and a challenge, intertwining state-of-the-art technology with strict regulatory standards.
- Technological Synergy: The alignment of AI tools with existing security protocols is fundamental. For example, evolving machine learning models to recognize new patterns of fraud not only bolsters our detection capabilities but also ensures that these systems are scalable and effective in the long run.
- Adaptive Algorithms: AI's real power in combating fraud lies in its remarkable adaptability. Keeping algorithms updated is essential to stay ahead of cybercriminals, ensuring that our defense mechanisms evolve as threats do.
- Regulatory Navigation: Adherence to regulations like GDPR and CCPA is not optional but mandatory, requiring AI solutions that are as compliant as they are effective. Implementing strategies such as data encryption and anonymization is vital to protect consumer information without compromising on fraud detection.
- Challenges: Embedding AI within cybersecurity frameworks demands a nuanced approach to data management, balancing automated decision-making with necessary human oversight. This balance ensures that AI tools support human judgment rather than attempting to replace it.
In the current landscape, where AI and synthetic identity fraud in e-commerce are increasingly prominent, prioritizing predictive analytics, fostering cross-platform defense networks, and investing in quantum-resistant technologies are critical strategies. These measures are not solely about preventing fraud; they are about ensuring the digital trust and privacy of consumers.
This pivotal moment in e-commerce challenges us to consider: How can we, as leaders in the field, effectively harness AI not just to counteract fraud but to advance digital security and trust?