How can AI Outsmart Zero-Day Vulnerabilities in the Retail Sector
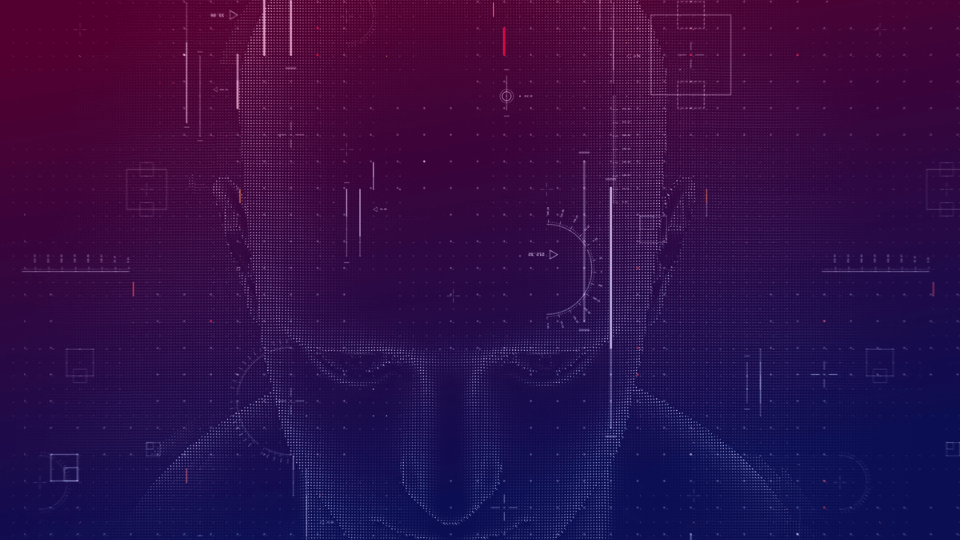
The explosive growth of e-commerce, projected by the National Retail Federation to climb by as much as 9.49% (CAGR 24-29), is dramatically outstripping traditional retail's growth. Yet, this expansion isn't without its pitfalls, especially in cybersecurity.
A zero-day vulnerability(ZVD) is a security flaw unknown to software developers until exploited. In retail, this might include unauthorized access to e-commerce platforms to steal customer data or exploiting Point of Sale (POS) systems for payment information theft
According to IBM's 2023 report, the global average cost of a breach has soared to $4.45 million, a 15% increase over the last three years, which emphasizes the economic toll of cybersecurity weaknesses in the e-commerce domain.
AI stands at the forefront of combating these cybersecurity threats. With its ability to predict potential attacks through analytics and identify anomalies in real time.
Businesses leveraging AI for cybersecurity are seeing substantial financial benefits, averting an average of $1.76 million in losses per incident. Furthermore, AI's capability to reduce the time needed to address breaches by 108 days, in comparison to traditional methods, highlights its indispensable role in securing the digital marketplace.
ZVDs
ZDV bypass traditional security measures, posing a direct risk to e-commerce platforms. Their nature makes early detection difficult, leaving e-commerce, with its extensive reach and large volume of transactions, especially exposed. The fallout from such breaches can stretch beyond financial losses to damage customer trust and brand reputation.
These vulnerabilities escape the notice of security teams until it's too late, rendering standard detection methods inadequate. As a result, the e-commerce industry, which thrives on digital trust, is at increased risk of financial and reputational harm. The challenge in detecting zero-day threats has led to a pivot towards heuristic and behavior-based detection methods.
In action:
- In early 2023, Darktrace's AI system made significant strides within an e-commerce setting by pinpointing anomalies through the behavioral patterns of each user and device. This technology played a pivotal role in thwarting a complex spear-phishing operation aimed at high-level executives, successfully preventing a financial crisis that could have cost upwards of $1.2 million.
- Shopify, taking a similar leap forward, harnessed machine learning to scrutinize account activities, resulting in a staggering 75% reduction in fraud incidents during the first few months of implementation. This achievement not only showcases the effectiveness of AI in detecting threats in real-time but also underscores its value in upholding strong security standards.
How can you integrate AI/ML for ZVDs?
A few steps that can be taken to implementing AI/ML into your cybersecurity strategy for ZVDs
- Advanced Anomaly Detection: Employ advanced AI algorithms for continuous monitoring and anomaly detection within retail IT ecosystems. Utilize deep learning to analyze transactional data, customer interactions, and endpoint activities for signs of unauthorized access or unusual patterns indicative of a ZVD exploit.
- Implement unsupervised machine learning models that dynamically adapt to the unique transactional behaviors of retail environments, detecting anomalies without predefined threat signatures.
- Predictive Threat Analysis: Integrate predictive analytics into the retail security stack to forecast potential ZVD attacks based on emerging trends in cyber threats and historical breach data.
- Use AI to perform sophisticated data mining on vast amounts of security logs and threat intelligence feeds, identifying subtle correlations that may predict future ZVD exploits.
- Customized AI-Driven Firewalls: Develop and deploy AI-enhanced firewalls tailored to the specific needs and threat vectors in the retail sector. These should dynamically adjust blocking rules and security policies in real-time based on AI-generated threat assessments.
- Incorporate natural language processing (NLP) to automatically analyze and interpret threat intelligence, adjusting firewall configurations to proactively counter identified vulnerabilities.
- Automated Patch Management: Leverage AI to streamline and optimize the patch management process, ensuring timely application of security patches to vulnerable systems and applications across retail networks.
- Apply reinforcement learning algorithms to prioritize patching efforts based on the criticality of assets and the potential impact of a ZVD, automating patch testing and deployment with minimal human intervention.
- Enhanced Incident Response: Utilize AI-driven Security Orchestration, Automation, and Response (SOAR) solutions for rapid containment and remediation of breaches. Tailor response playbooks to the retail context, ensuring customer data protection and business continuity are prioritized.
- Integrate machine learning with incident response protocols to automate the analysis of breach impacts, coordinate response actions across disparate retail systems, and optimize recovery processes.
But there is always a question of implementation challenges around ethics, data and bottlenecks.
Solving implementation challenges:
Integrating AI into cybersecurity brings to the forefront complex challenges such as data privacy and algorithm bias, necessitating a sophisticated yet ethical approach to solutions.
- Deep Dive into Differential Privacy: Shopify's use of differential privacy exemplifies a sophisticated approach to protecting user data. By applying mathematical techniques to anonymize individual transactions within its datasets, Shopify adds 'noise' to the data. This process ensures that while the data becomes slightly less precise due to this 'noise,' it remains impossible to trace back to any personal information. Consequently, Shopify can glean valuable insights for fraud detection without compromising the privacy of individual customers.
- Detailing Algorithm Bias Mitigation: Alibaba's effort to diversify its training datasets showcases a proactive approach to enhancing the fairness of AI algorithms. By incorporating a broader range of data, including transactions from various demographics and geographies, Alibaba ensures its AI systems are exposed to a wide spectrum of user behaviors. This diversity in training data helps minimize biases, leading to a 20% improvement in the accuracy of its fraud detection mechanisms.
The initiatives of Shopify and Alibaba demonstrate quantifiable benefits, with Shopify’s differential privacy technique notably improving fraud detection while adhering to strict data protection standards like GDPR and CCPA. Similarly, Alibaba's enriched datasets not only boost algorithm accuracy but also enhance customer trust, evidenced by improved satisfaction scores.
In conclusion
The stakes of neglecting such advancements are high. Take Home Depot’s case. Cyber attackers exploited a zero-day flaw in the company's payment systems to install custom malware. This breach compromised the payment information of approximately 56 million customers, making it one of the largest in retail history. . This incident underscores the critical financial, trust, and reputation repercussions that can arise from inadequate cybersecurity measures. Therefore, the call to action is clear: e-commerce platforms must proactively integrate AI into their ZVD strategy to safeguard their operations, reputation, and, most importantly, their customers.