Banking Resilience: A Response to Third-Party Vulnerabilities
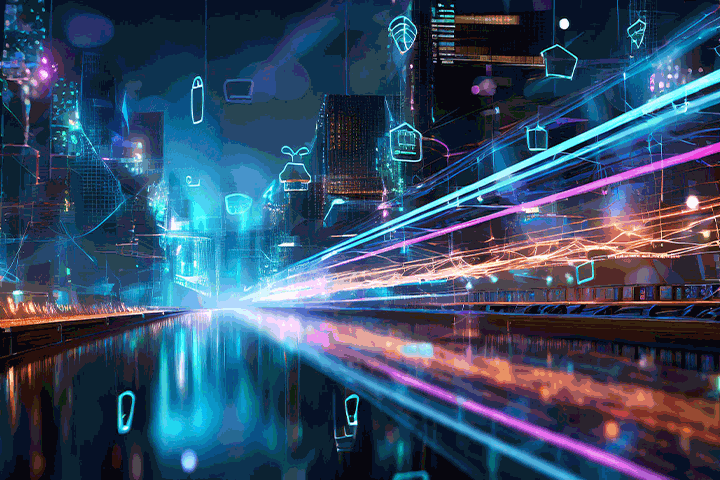
The 2020 SolarWinds cyberattack spotlighted critical vulnerabilities within the banking sector, signaling an immediate need for enhanced third-party risk assessments. By exploiting software weaknesses, the incident thrust the industry into heightened regulatory focus and necessitated an upgrade in risk management strategies. The Ponemon Institute's 2021 analysis revealed the banking sector as the primary victim of breach-related expenses, incurring an average cost of $5.85 million per breach. This figure highlights the profound financial implications and the consequent effects on customer trust and regulatory adherence, begging the importance of the third party risks.
Another notable event was what happened in December 2013 with Target. In this incident, hackers were able to gain unauthorized access to Target's network through credentials stolen from a third-party vendor, specifically an HVAC (Heating, Ventilation, and Air Conditioning) contractor. Once inside the network, the attackers deployed malware to Target's point-of-sale (POS) systems and successfully exfiltrated the credit and debit card data of approximately 40 million customers, along with the personal information of up to 70 million individuals.
This incident was a wake-up call for banks, pushing them to move away from manual, checklist-based evaluations towards a more robust, technology-centric approach for managing third-party risks.
Post-2008 Technological Advancements
In the wake of digital transformation post-2008, the banking sector's approach to third-party cybersecurity vendor risk evolved significantly:
- Third-Party Software and Services: Banks' reliance on external software and services for online and mobile banking necessitated enhanced security vetting of vendors to guard against vulnerabilities, inspired by incidents like the Target breach.
- Data Storage and Management: Transitioning to cloud computing required banks to ensure third-party cloud providers adhered to strict data protection measures and financial regulations, emphasizing encryption and access control to safeguard customer data.
- Payment Processing Systems: The integration of third-party payment processors for mobile transactions highlighted the need for robust security protocols, including end-to-end encryption, to prevent financial fraud.
- The Digital Shift’s Broader Impact: Bank of America - pushing the envelope in Digital transformation. This strategy, although beneficial for streamlining operations, also introduced banks to new cybersecurity challenges, such as a broader attack surface and the complexity of securing cloud environments. A significant rise in supply chain attacks, with a 75% increase noted by Symantec, highlights the growing threats.
Regulatory Landscape Adjustments
Adjusting to digital advancements and their risks has been heavily influenced by regulations like the GDPR and NYDFS Cybersecurity Regulation. These regulations set strict guidelines for incident response and regular audits of third-party vendors, urging banks to adopt more thorough compliance and risk management strategies.
Through learning from past breaches, complying with stringent regulatory requirements, and leveraging new technologies, the banking sector is undergoing a remarkable transformation today, sought with transformational bottlenecks, ethical questions and sheer engineering grit.
AI-Driven Models for Risk Management
Take Google Cloud's Anti Money Laundering AI (AML AI), for instance. Its implementation by HSBC showcases a leap in technology's ability to pinpoint accurate anomalies—detecting 2-4 times more legitimate threats and reducing false positives by over 60%. This isn't just a marginal improvement; it's a transformation in how financial institutions can sift through vast data to identify real risks, marking a shift to smarter, data-driven decision-making in third-party risk management.
Beyond risk management, AI's role in banking has diversified. Morgan Stanley’s use of OpenAI-powered chatbots, and Bank of America and Wells Fargo's deployment of conversational AI, demonstrate the technology's breadth. These AI applications do more than just automate tasks; they provide personalized, data-informed insights and support to customers. The result is a smoother operational process and a superior customer experience, highlighting AI’s ability to serve dual purposes—operational efficiency and customer satisfaction.
Ethical Considerations in AI
However, integrating AI into banking isn't without its ethical dilemmas, including data privacy, bias in algorithms, and the need for transparency. The IEEE’s Ethically Aligned Design principles offer a framework to address these challenges, emphasizing ethical responsibility in the development and deployment of AI technologies. By adhering to such guidelines, banks can ensure their AI tools are not only effective but also fair and respectful of privacy, maintaining the trust of their customers.
Challenges and questions:
Let's look at the broader strategy and challenges faced by banks incorporating AI for third-party risk management.
The move towards AI reflects a necessary shift, aiming for the agility seen in fintech while meeting strict regulatory standards- Integrating AI is far from straightforward. Banks grapple with making their AI vision compatible with existing legacy systems. This challenge is multi-faceted, involving outdated technologies, unclear AI strategies, and insufficient data infrastructure. Citibank's response, focusing on an ethics framework for AI, suggests a comprehensive strategy that's about more than just tech upgrades. It includes fostering an innovative culture and ensuring AI systems work seamlessly with existing infrastructures.
Tackling these issues demands a strategic, step-by-step approach to AI adoption. Critical actions include enhancing data quality, filling the skills gap, achieving smooth integration of AI with legacy systems, and managing the ethical and regulatory landscape effectively. This plan lays the groundwork for advancing towards predictive analytics and machine learning, aiming at proactive risk identification and mitigation.
Looking ahead, the role of AI in third-party risk management is set to grow. With predictive analytics and machine learning becoming more central, continuous innovation becomes crucial - prompting the question: How will these advancements alter our confidence in the banking sector's capacity to guard against new and evolving threats?