AI and Legacy Banking Systems: Going from Ransom to resilience.
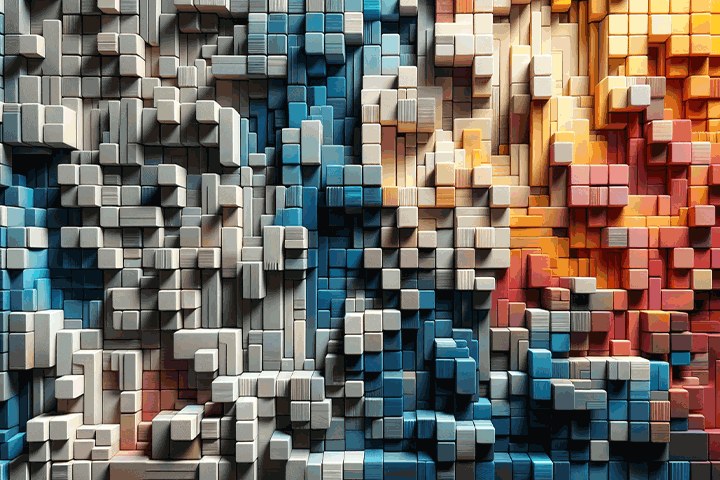
Introduction:
In 2021, the security landscape of the global financial sector faced a significant test when JBS Bank fell victim to a ransomware attack, leading to a substantial $11 million ransom payment. This event serves as a stark reminder of the vulnerabilities present within traditional banking systems, highlighting a pivotal moment in the realm of financial cybersecurity.
Against this backdrop, Artificial Intelligence (AI) has for years, especially the last 2 years, stood out not just as a mere tool but as a game-changer in crafting cyberdefense strategies.
The Context of Banking & Security:
Digital Transformation and Emerging Threats: The introduction of the internet and online banking in the 1990s brought forth new cybersecurity challenges. The early 2000s marked the rise of phishing attacks designed to trick individuals into revealing confidential information.
Regulatory Responses to Growing Cyber Threats: The Basel II and III frameworks were developed to bolster the financial industry's defense against operational risks, including cyber threats. The Payment Card Industry Data Security Standard (PCI DSS) was established to ensure the security of credit and debit card transactions.
Sophistication of Cyber Threats: The 2010s witnessed the emergence of advanced persistent threats (APTs) and state-sponsored attacks targeting financial institutions.
Significant incidents include the 2013 attack on South Korean banks and the 2016 cyber heist at the Bangladesh Central Bank.
The Rise of AI: The adoption of AI and machine learning for anomaly detection and fraud prevention has started to gain momentum. IBM Watson's deployment for cognitive threat intelligence and anomaly detection represents a significant step forward in integrating AI into financial cybersecurity strategies.
The Integration of AI in Banking Cybersecurity
The past ten years have seen banks embarking on a digital journey, with AI emerging as a cornerstone in fortifying cybersecurity defenses. This transformation has not only bolstered security measures but also demanded a flawless melding with the banks' existing infrastructure.
Machine Learning Algorithms:
Through Supervised Learning, banks educate models on past transactions, effectively distinguishing between fraudulent and legitimate activities.
Unsupervised Learning shines by spotting irregularities in transactions, flagging potential fraud without the need for preset categories.
A notable implementation can be seen with Citibank. The institution cleverly married machine learning with its established systems via bespoke middleware. This fusion has significantly enhanced fraud detection by enabling the analysis of transaction data in real time.
Natural Language Processing (NLP):
NLP seamlessly integrates into the cybersecurity framework, automating the identification of indicators of financial crimes from unstructured data, thus sharpening threat intelligence.
In practice, NLP proved its worth by uncovering a phishing attempt aimed at Wells Fargo customers, through a detailed analysis of complaints received via emails and social media, enabling swift preventative measures.
Blockchain:
The technology stands out by employing an immutable ledger and smart contracts, ensuring transactions are secure and verifiable. JPMorgan Chase embarked on a blockchain experiment, integrating it alongside traditional ledgers for less risky transactions and ensuring seamless interoperability with existing systems through APIs.
Embracing these AI technologies not only addresses the present cybersecurity threats but also scales to confront future challenges. Initiatives like the FS-ISAC exemplify the power of collaboration, sharing vital intelligence and best practices, thus strengthening the collective defense mechanism of the financial sector.
In the Trenches: Success stories
Let’s take a closer look at how Deutsche Bank and Barclays are leading the way in cybersecurity and transaction safety through the practical application of AI and blockchain technologies.
Deutsche Bank's Leap into AI-Driven Fraud Detection
At Deutsche Bank, the integration of AI into their cybersecurity framework has significantly amped up their fraud detection capabilities, fostering a deeper trust among customers and ensuring stringent compliance. This move is a vivid illustration of the bank's progression towards digital sophistication, with an acute emphasis on halting fraud as it happens.
- The Challenge: Meshing AI with the demands of real-time processing was no small feat. The bank had to navigate through a maze of technical and cultural adjustments to achieve the kind of precision that can pinpoint fraud in milliseconds.
- The Solution: The breakthrough came with the development of a microservices-based API, a solution that allowed Deutsche Bank to flexibly adapt AI for spotting fraud patterns without missing a beat in system performance.
- The Outcome: This strategic integration led to a 25% drop in fraudulent transactions. Particularly striking was the discovery of a complex fraud scheme that had slipped under the radar, showcasing the advanced detection capabilities of the AI solution.
- Lessons Learned: The journey highlighted the necessity of an ongoing refinement of AI algorithms, keeping pace with the ever-evolving landscape of fraud to maintain a strong defensive stance.
Barclays Banks on Blockchain for Enhanced Security
Barclays turned to blockchain technology as a concrete step towards securing transactions, showcasing its forward-thinking approach to minimizing operational risks and enhancing transparency in transactions.
- The Challenge: Merging blockchain with the bank's existing frameworks presented a unique set of challenges, from ensuring seamless interoperability to meeting stringent regulatory standards. Convincing the bank's internal skeptics of the scalability and compliance benefits was crucial.
- The Solution: The bank initiated pilot projects focusing on a mix of internal and selective external transactions to test the waters of blockchain integration, making use of smart contracts to streamline compliance and settlements.
- The Outcome: The adoption of blockchain technology not only sped up transactions but also brought about a notable improvement in the efficiency of cross-border payments, signaling a shift towards the digital banking of the future.
- Lessons Learned: This venture marked a significant cultural shift within Barclays, acknowledging the untapped potential of blockchain and laying the groundwork for future technological exploration.
Charting the Course: Navigating Implementation Challenges
Embedding AI within the intricate web of legacy banking systems requires a thoughtful approach to tackle issues around data compatibility, system architecture, and privacy.
- Data Compatibility: Leveraging data transformation tools and crafting comprehensive data governance frameworks are essential steps towards syncing AI systems with the existing banking databases.
- System Architecture Adjustments: Examples like HSBC showcase how banks have adeptly navigated architectural changes, cleverly balancing hardware updates with cloud-based AI to keep infrastructural tweaks to a minimum.
- Privacy: Cutting-edge approaches like federated learning are pushing the envelope in data privacy, enabling the training of AI models across decentralized systems without the need for direct data sharing.
- Strategic Rollout: A step-by-step integration strategy, supported by measurable KPIs for pilot assessments, encourages widespread acceptance within the organization and eases the transition, ensuring that everyone is on board and aligned with the new technological direction.
Some steps for CISOs:
- Audit and Assess: Begin with comprehensive audits to uncover vulnerabilities. This crucial step paves the way for targeted AI enhancements.
- Customize AI Solutions: Matching AI technologies with specific security challenges ensures a defense as unique as the threats it counters.
- Develop a Strategic Roadmap: Prioritize initiatives based on their potential to bolster security. A clear plan is essential for navigating the complexities of AI integration.
Concluding Thoughts
AI has emerged as a formidable defender against dynamic threats to banking security. While integrating AI poses challenges, a strategic framework and a vision for the future prepare us for a secure banking environment.
As we stand at this critical juncture, the decisions on technology adoption and strategic direction will define our resilience against future threats. This path requires foresight and a dedication to innovation.
How will our choices today shape the security and trustworthiness of banking for generations to come?